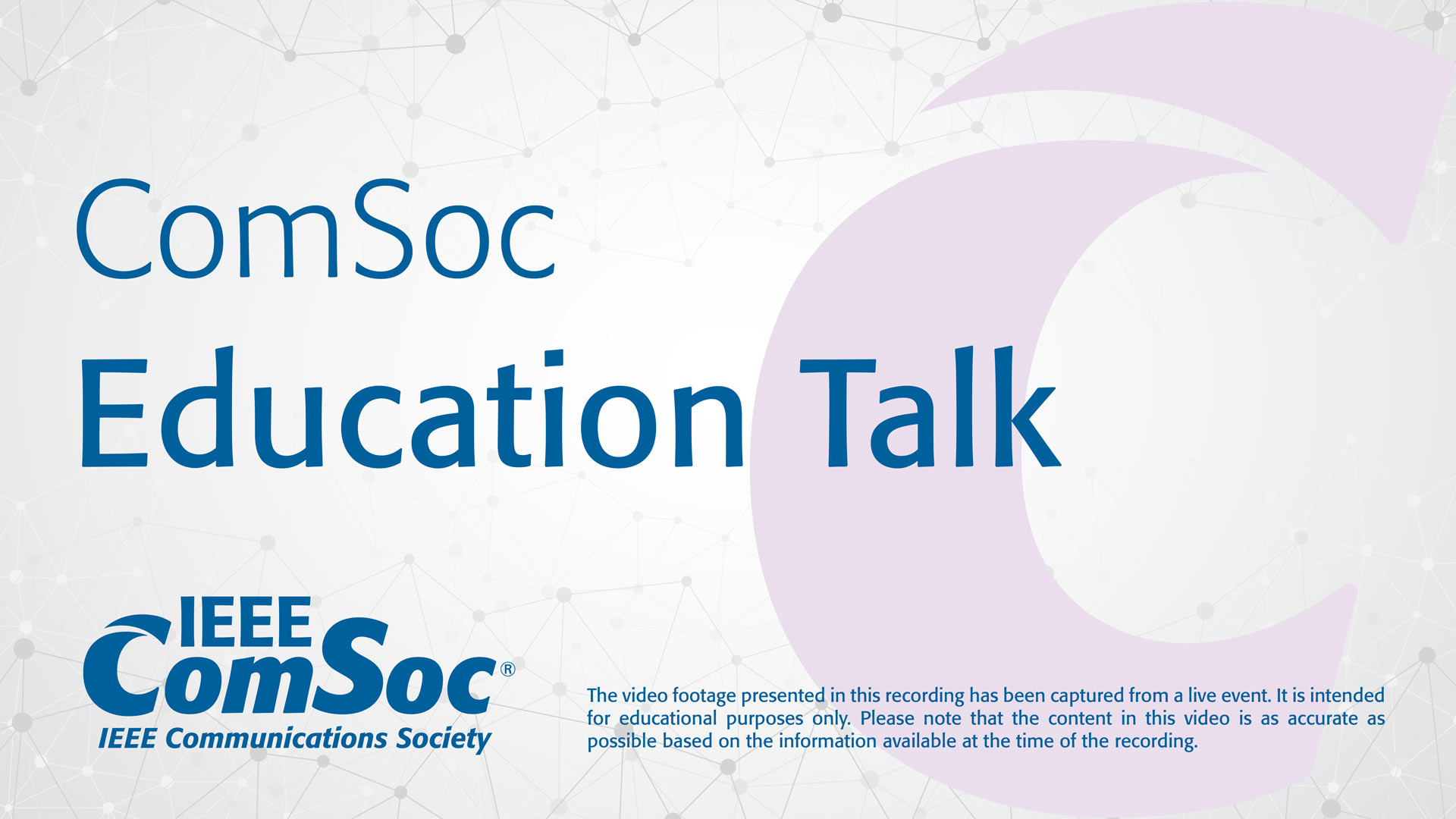
Description
Confidential computing based on Fully Homomorphic Encryption (FHE) is gaining attention. FHE enables arbitrary computation on encrypted data and thus enables the privacy of users' data. Ever since the first FHE scheme based on lattice was proposed by Craig Gentry in the year 2009, many FHE schemes are designed. Some of prominent FHE schemes BGV, CKKS, TFHE . In the meantime, many open source libraries for FHE schemes such as HElib SEAL, HEAAN, PALiSADE, etc. are also developed. This enabled to realize some of the applications in the area of data analytics and private AI (private inference) based on FHE. However, due to considerable computation/memory overhead, the performance of FHE schemes is slow of the order of 6 when compared to computation on plaintext data. Hence to address this, there are several research works devoted to accelerating FHE schemes. As part of this talk, we cover, optimizations in FHE for realizing privacy preserving machine learning applications in an efficient manner.
Presenters
Rajan M A
ComSoc Member Price
$0.00
IEEE Member Price
$4.99
Non-Member Price
$9.99