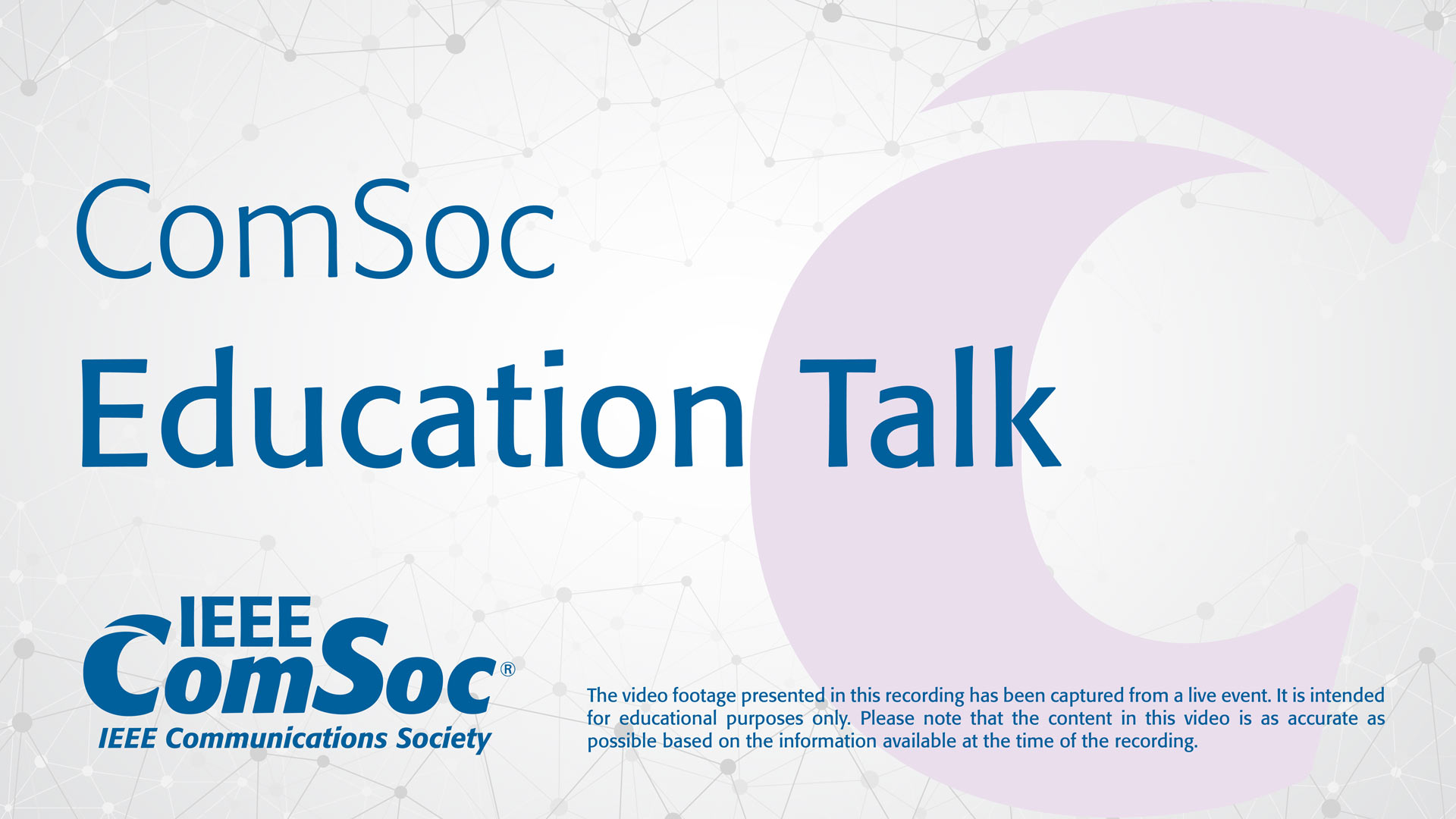
Description
Machine learning (ML) and AI will play a key role in the development of 6G networks. Network virtualization and network softwarization solutions in 5G networks can support data-driven intelligent and automated networks to some extent and this trend will grow in 5G-advanced networks. Radio access network algorithms and radio resource management functions can exploit network intelligence to fine-tune network parameters to reach close-to-optimal performance in 5G networks. In 6G networks, network intelligence is envisioned to be end-to-end, and air interface is envisioned to be AI-native. The user equipment (UE) devices need to be smarter, environment and context-aware, and capable of running ML algorithms. With these capabilities on end devices, federated learning is envisioned to be one of the promising solutions that can solve the scalability and trust issues in distributed learning. This talk will focus on the main practical challenges in developing machine learning solutions in 5G use cases, related 3GPP standardization activities, and emphasize with a case study how the deployment of these solutions is much harder in a real network as compared to theoretical performance evaluation. Furthermore, the use of federated learning in wireless networks is motivated by providing example use case examples; and challenges in the use of federated learning solutions in 6G networks are explained.
Presenters
Majid Butt
ComSoc Member Price
$0.00
IEEE Member Price
$4.99
Non-Member Price
$9.99