Maziar Nekovee, University of Sussex and Quantrom Technologies Ltd, UK, Dehao Wu, University of Sussex, UK, Yue Wang, Samsung R&D Institute, UK, Sachin Sharma, National College of Ireland, Ireland, Avishek Nag, University College Dublin, Ireland
Published: 15 Aug 2019

CTN Issue: August 2019

A note from the editor:
Now, given the ever-increasing importance of artificial intelligence, machine learning and automation in the digital transformation of our society, economy and industry, future mobile network is not an exception! To put things simple, artificial intelligence and machine learning enabled mobile networks including infrastructure and services will be able to observe, learn and adapt their operations according to environment and user requirement following the cognitive cycle and drive models and theoretic approaches, protocols design and infrastructure optimisation and thereby guarantee large scale automation, systems and services requirements and leverage complex distributed architectures.
In this article, Maziar Nekovee, Dehao Wu, Yue Wang, Sachin Sharma, and Avishek Nag present how artificial intelligence and machine learning can facilitate future of mobile networks. Some very interesting and futuristic use cases, namely, AI for infrastructure, AI for PHY design and AI for spectrum access or sharing are presented. Key idea here is to demonstrate the realistic integration of artificial intelligence and machine learning in future mobile networks a.k.a. 6G network and paving the way towards solving the open challenges and complex issues in networks.
Enjoy these fascinating set of case studies and as always, your comments are most welcome.
Editor

AI for Mobile Networks
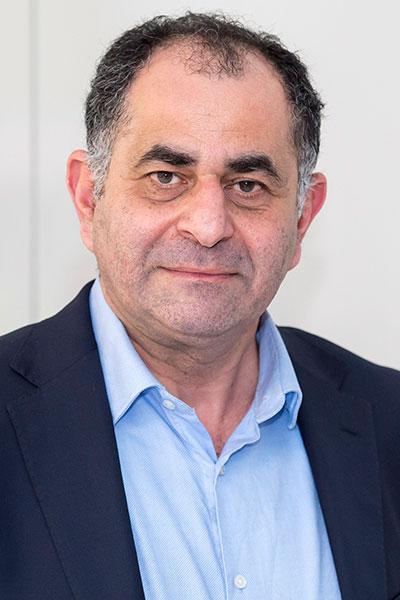
Maziar Nekovee
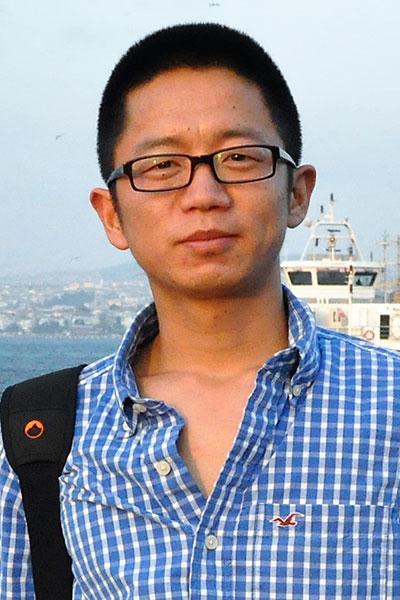
Dehao Wu
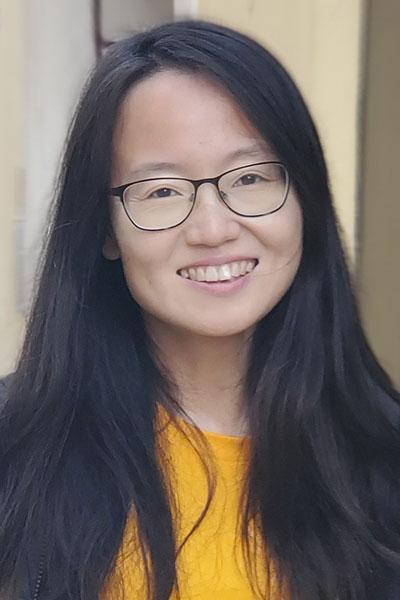
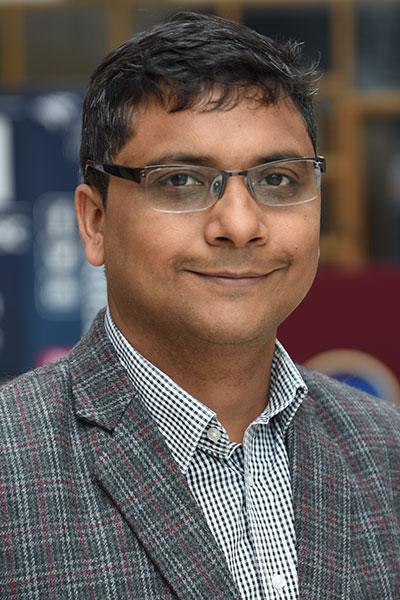

5G, the new generation of mobile communication systems with its well-known ITU 2020 triangle of new capabilities, which not only include ultra-high speeds but also ultra-low latency, ultra-high reliability, and massive connectivity promise to expand the applications of mobile communications to entirely new and previously unimagined “vertical industries” and markets such as self-driving cars, smart cities, industry 4.0, remote robotic surgery, smart agriculture, and smart energy grids. The mobile communications system is already one of the most complex engineering systems in the history of mankind. As 5G network penetrates deeper and deeper into the fabrics of the 21st century society, we can also expect an exponential increase in the level of complexity in design, deployment, and management of future mobile communication networks which, if not addressed properly, have the potential of making 5G the victim of its own early successes.
Breakthroughs in Artificial Intelligence (AI) and Machine Learning (ML), including deep neural networks and probability models, are creating paths for computing technology to perform tasks that once seemed out of reach. Taken for granted today, speech recognition and instant translation once appeared intractable, and the board game ‘Go’ had long been regarded as a case testing the limits of AI. With the recent win of Google’s ‘AlphaGo’ machine over world champion Lee Sedol — a solution considered by some experts to be at least a decade further away — was achieved using a ML-based process trained both from human and computer play. Self-driving cars are another example of a domain long considered unrealistic even just a few years ago — and now this technology is among the most active in terms of industry investment and expected success. Each of these advances is a demonstration of the coming wave of as-yet-unrealized capabilities. AI, therefore, offers many new opportunities to meet the enormous new challenges of design, deployment, and management of future mobile communication networks in the era of 5G and beyond, as we illustrate below using a number of current and emerging scenarios.
Network Function Virtualization Design with AI
Network Function Virtualization (NFV) [1] has recently attracted telecom operators to migrate network functionalities from expensive bespoke hardware systems to virtualized IT infrastructures where they are deployed as software components. A fundamental architectural aspect of the 5G network is the ability to create separate end-to-end slices to support 5G’s heterogeneous use cases. These slices are customised virtual network instances enabled by NFV. As the use cases become well-defined, the slices need to evolve to match the changing users’ requirements, ideally in real time. Therefore, the platform needs not only to adapt based on feedback from vertical applications, but also do so in an intelligent and non-disruptive manner. To address this complex problem, we have recently proposed the 5G NFV "microservices" concept, which decomposes a large application into its sub-components (i.e., microservices) and deploys them in a 5G network. This facilitates a more flexible, lightweight system, as smaller components are easier to process. Many cloud-computing companies, such as Netflix and Amazon, are deploying their applications using the microservice approach benefitting from its scalability, ease of upgrade, simplified development, simplified testing, less vulnerability to security attacks, and fault tolerance [6]. Expecting the potential significant benefits of such an approach in future mobile networks, we are developing machine-learning-aided intelligent and optimal implementation of the microservices and DevOps concepts for software-defined 5G networks. Our machine learning engine collects and analyse a large volume of real data to predict Quality of Service (QoS) and security effects, and take decisions on intelligently composing/decomposing services, following an observe-analyse-learn- and act cognitive cycle.
We define a three-layer architecture, as depicted in Figure 1, composing of service layer, orchestration layer, and infrastructure layer. The service layer will be responsible for turning user’s requirements into a service function chain (SFC) graph and giving the SFC graph output to the orchestration layer to deploy it into the infrastructure layer. In addition to the orchestration layer, components specified by NFV MANO [1], the orchestration layer will have the machine learning prediction engine which will be responsible for analysing network conditions/data and decompose the SFC graph or network functions into a microservice graph depending on future predictions. The microservice graph is then deployed into the infrastructure layer using the orchestration framework proposed by NFV-MANO.

Physical Layer Design Beyond-5G with Deep-Neural Networks
Deep learning (DL) based auto encoder (AE) has been proposed recently as a promising, and potentially disruptive Physical Layer (PHY) design for beyond-5G communication systems. DL based approaches offer a fundamentally new and holistic approach to the physical layer design problem and hold the promise for performance enhancement in complex environments that are difficult to characterize with tractable mathematical models, e.g., for the communication channel [2]. Compared to a traditional communication system, as shown in Figure 2 (top) with a multiple-block structure, the DL based AE, as shown in Figure 2 (bottom), provides a new PHY paradigm with a pure data-driven and end-to-end learning based solution which enables the physical layer to redesign itself through the learning process in order to optimally perform in different scenarios and environment. As an example, time evolution of the constellations of two auto encoder transmit-receiver pairs are shown in Figure 3 which starting from an identical set of constellations use DL-based learning to achieve optimal constellations in the presence of mutual interference [3].
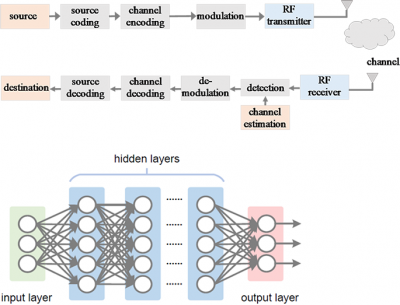

Spectrum Sharing with AI
The concept of cognitive radio was originally introduced in the visionary work of Joseph Mitola as the marriage between wireless communications and artificial intelligence, i.e., wireless devices that can change their operations in response to the environment and changing user requirements, following a cognitive cycle of observe/sense, learn and act/adapt. Cognitive radio has found its most prominent application in the field of intelligent spectrum sharing. Therefore, it is befitting to highlight the critical role that AI can play in enabling a much more efficient sharing of radio spectrum in the era of 5G. 5G New Radio (NR) is expected to support diverse spectrum bands, including the conventional sub-6 GHz band, the new licensed millimetre wave (mm-wave) bands which are being allocated for 5G, as well as unlicensed spectrum. Very recently 3rd Generation Partnership Project (3GPP) Release-16 has introduced a new spectrum sharing paradigm for 5G in unlicensed spectrum. Finally, both in the UK and Japan the new paradigm of local 5G networks are being introduced which can be expected to rely heavily on spectrum sharing. As an example of such new challenges, the scenario of 60 GHz unlicensed spectrum sharing is shown in Figure 4(a), which depicts a beam-collision interference scenario in this band. In this scenario, multiple 5G NR BSs belonging to different operators and different access technologies use mm-wave communications to provide Gbps connectivity to the users. Due to high density of BS and the number of beams used per BS, beam-collision can occur where unintended beam from a “hostile” BS can cause server interference to a user. Coordination of beam-scheduling between adjacent BSs to avoid such interference scenario is not possible when considering the use of the unlicensed band as different BS operating in this band may belong to different operators or even use different access technologies, e.g., 5G NR versus, e.g., WiGig or Multifire. To solve this challenge, reinforcement learning algorithms can successfully be employed to achieve self-organized beam-management and beam-coordination without the need for any centralized coordination or explicit signalling [4]. As 4(b) demonstrates (for the scenario with 10 BSs and cell size of 200 m) reinforcement learning-based self-organized beam scheduling (algorithms 2 and 3 in the Figure 4(b)) can achieve system spectral efficiencies that are much higher than the baseline random selection (algorithm 1) and are very close to the theoretical limits obtained from an exhaustive search (algorithm 4), which besides not being scalable would require centralised coordination.
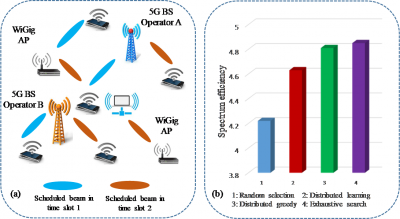
Figure 4: Spectrum sharing scenario in unlicensed mm-wave spectrum (left) and system spectral efficiency of 10 BS deployment (right). Results are shown for random scheduling (algorithm 1), two versions of ML-based schemes (algorithms 2 and 3) and theoretical limit obtained from exhaustive search in beam configuration space (algorithm 4).
Conclusions
In this article, we presented few case studies to demonstrate the use of AI as a powerful new approach to adaptive design and operations of 5G and beyond-5G mobile networks. With mobile industry heavily investing in AI technologies and new standard activities and initiatives, including ETSI Experiential Networked Intelligence ISG [5], the ITU Focus Group on Machine Learning for Future Networks Including 5G (FG-ML5G) and the IEEE Communication Society’s Machine Learning for Communications ETI are already actively working on harnessing the power of AI and ML for future telecommunication networks, it is clear that these technologies will play a key role in the evolutionary path of 5G toward much more efficient, adaptive, and automated mobile communication networks. However, with its phenomenally fast pace of development, deep penetration of Artificial Intelligence and machine-learning may eventually disrupt the entire mobile networks as we know it, hence ushering the era of 6G.
References
- ETSI ISG for NFV, “ETSI GS NFV 002: Network Functions Virtualisation (NFV); Architectural Framework,” http://www.etsi.org/deliver/etsi_gs/NFV/001_099/002/01.02.01_60/gs_NFV002v010201p.pdf; January 2015.
- T. J. O’Shea and J. Hoydis, “An introduction to deep learning for the physical layer,” IEEE Transactions on Cognitive Communications and Networking, vol. 3, no. 4, pp. 563-575, December 2017.
- D. Wu, M. Nekovee, Y. Wang, An adaptive deep learning based auto encoder for the interference channel, available from https://arxiv.org/abs/1902.06841
- M. Nekovee, Y. Qi, Y. Wang, Distributed beam-scheduling for multi-RAT coexistence in mm-wave 5G Networks, Proc. IEEE PIMRC 2016.
- ETSI ISG White Paper No. 22 “Improved Operator Experience through Experiential Networked Intelligence (ENI)”, October 2017.
- S. Sharma, N. Uniyal, B. Tola, Y. Jiang, “On monolithic and microservice deployment of network functions”, Proc. IEEE Conference on Network Softwarization (NetSoft), pp. 388-395, 2019
Statements and opinions given in a work published by the IEEE or the IEEE Communications Society are the expressions of the author(s). Responsibility for the content of published articles rests upon the authors(s), not IEEE nor the IEEE Communications Society.
Comments
I enjoyed this article, and I believe that it describes a path forward in network design that is reasonable in the short term. If we look beyond 6G, 7G, and so on, our telecommunications networks will evolve to look like the neurological networks of the brain. These networks will be orders of magnitude more powerful, will exhibit real intelligence and not artificial intelligence, and will require a multidisciplinary approach to design developed by a team consisting of electrical and computer engineers, mechanical engineers, chemical engineers, life scientists, and neuroscientists. See my articles in The Conversation (theconversation.com) to get a feeling for where things are headed. The IEEE Communications Society would really be exercising leadership if it created an avenue at conferences and in journals for those who share this vision.
Submitted by sdmorgera@usf.edu on 10 September 2019
A great read.
Submitted by ee18s014@smail… on 28 August 2019