Ahmed Bader, Tareq Al-Naffouri, Mohamed-Slim Alouini, Hebah A. ElGibreen, Abdulkareem Adinoyi, Salah S. Almofleh, and Rakan Alseghayer
Published: 5 Jan 2024

CTN Issue: January 2024
A note from the editor:
We wish to thank our readers for their support during 2023, we hope this continues and grows in 2024. During the year we have tried to offer a variety of subjects to our readers and have introduced the Podcast feature into CTN, I encourage you to take a look and provide your feedback. We are always on the lookout for contributions, feel free to contact us if you wish to propose or send us a contribution. The editorial board wishes everyone a wonderful holiday season and a healthy and rewarding new year.
To end 2023 and start 2024, we share an article where the authors discuss why Open RAN might find a home in smart cities. In reading recent press releases and general communications news, we can see an increased number of announcements about Open RAN based private/enterprise networks. Smart cities have always been a shiny example of where automation and IoT technologies can help improve the way in which we live, and things are managed in a city, so is Open RAN the right choice for Smart Cities?
Miguel Dajer, CTN Editor-in-Chief
Open RAN … Unlocking New Heights for Smart Cities



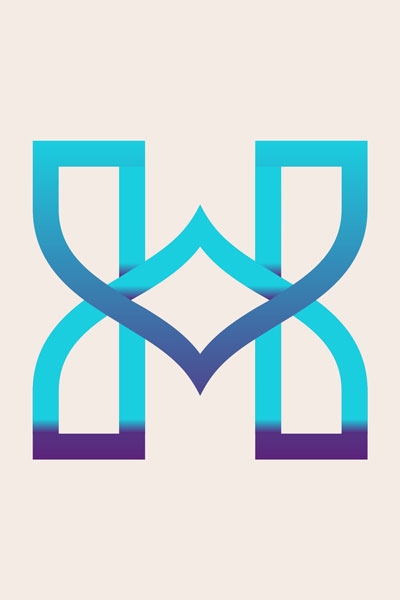


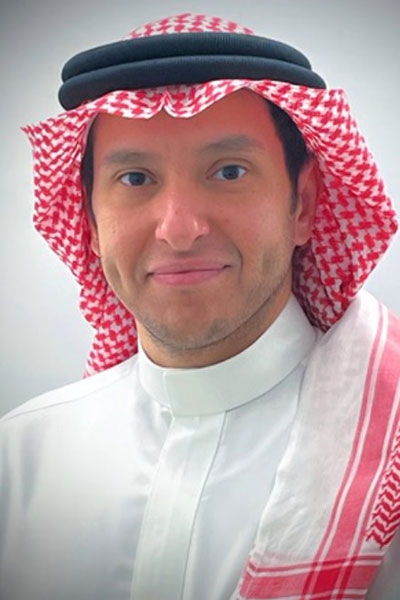
An Ill-Fated Approach
The need for making our cities smarter is evolving [NEOM]. In the quest for improving the livability, efficiency and sustainability of cities, all sorts of systems are being rolled out, adding new pieces of equipment, cameras, sensors, and infrastructure to the mix. In a country such as Saudi Arabia, where 85% of the population lives in a city [SUSC], the evolution and transformation towards smart city paradigms may therefore prove unnecessarily costly. However, it is straightforward to recognize that there is one truly ubiquitous and scalable system, which is underutilized, i.e. mobile networks!
For instance, why are cameras the dominant technology for crowd management [CAMC]? On the other hand, why is new Bluetooth and Wi-Fi gear typically needed for footfall analytics [WBLE]? Similarly, why are we so dependent on using mobile app data to obtain commute behavioral analytics [MAPP]? At the same time, why do we not yet have an accurate system in place for estimating greenhouse gas emissions, for example from transportation, in a city [GHGC]? Given the obvious ability of mobile networks to furnish this data, why is it not happening at scale? In fact, why is the mobile infrastructure being primarily used only for connectivity [CONN]?.
Yes, COVID Was There
Back in 2020/2021, amid the intensity of the COVID-19 crisis, our team began contemplating how to extract useful data from mobile networks. At the time, there was an urgent need for contact tracing solutions, to support health services and individuals. Our hypothesis was that using mobile networks would avoid the use of intrusive mobile apps while achieving the same results [COVA]. We then quickly realized that an opportunity had always existed, in properly using mobile network data to solve real-world problems – there was a goldmine in plain sight, waiting to be excavated. The goldmine is radio access network (RAN) data. In the early months of 2021, our team successfully demonstrated [LUCA] that RAN data (also referred to as radio traces in technical literature) are as informative as the accelerometer and gyroscope inside a smartphone. With RAN data, you can tell if two people are next to each other or not, how many people are riding a bus or train, whether someone is biking, walking, riding a car, or simply standing still [LUCA].
Open RAN Coming to Rescue
By the end of 2021, we were able to develop artificial intelligence (AI) models that ingest RAN data and produce all sorts of mobility analytics like the ones mentioned above [LUCA]. At a later stage, we even started experimenting with AI models that can distinguish between a sidewalk bot and a drone and estimate the speed and altitude of the latter. Our initial AI models were validated in the lab and in controlled field experiments, giving us confidence to test them on an actual 5G network. We began running proof-of-concept (PoC) experiments (Figure 1) in the Eastern Province of Saudi Arabia in early 2022 [EAST].
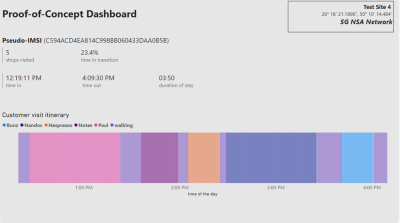
While these PoCs were quite promising, they uncovered a less explicit gap, but a serious one. Today, mobile networks are built end-to-end using a monolithic architecture, offered by a handful of vendors. The task of exposing the RAN data needed by our AI model, extracting it, and feeding it to the models, is both cumbersome and non-systematic. However, the timing could not be better! A paradigm shift in how mobile networks are built has been underway since 2018, i.e., Open RAN (O-RAN). The industry had been moving towards making mobile networks more open, softwarized, cloudified, and virtualized – exactly what is needed to accomplish our goals and drive our mission forward.
Multiple live O-RAN trials have been conducted in our region to explore the supply chain landscape, use cases, and value proposition of the technology [GCCO]. stc and other regional mobile network operators took to the field in a collaborative effort aimed at ensuring effective cross-migration of industry knowledge and expertise within the region. While the outcomes were largely promising, the trial also reaffirmed the conviction that further evolution of the hardware and software is required to address use cases that may not necessarily relate to pure connectivity and content services.
Non-Conventional Wisdom
The conventional wisdom behind Open RAN is breaking vendor lock-in, access to best-of-breed software and hardware, with the goal of eventually improving total cost of ownership (TCO). An essential element in the Open RAN stack is the RAN intelligent controller (RIC). The dominant view of RIC is that it is a tool for “closed-loop” value creation. This involves extracting data from the Open RAN system and employing it to improve the network’s energy efficiency and reduce the amount of labor needed for network maintenance. This provides a pathway for Open RAN technology to also reduce an operator’s OPEX. This approach is referred to as closed-loop (Figure 2) because it extracts data and then communicates control actions back to the RAN.

On the flip side of the coin, open-loop value creation involves extracting data from various layers, levels, and touchpoints within the RAN stack. The data is run in previously-ratified AI models to generate analytics work streams. We can package the associated outputs into business intelligence (BI) and decision intelligence (DI) insights used in a number of market verticals. This presents the telco with opportunities to unlock new value, and address a wide range of smart and cognitive city applications at scale.
Practical Use Cases
As part of our customer and market discovery exercise, we came across a number of use cases across multiple smart city verticals, including mega-events, large-scale retail spaces, and public transportation (Figure 3). The latter is probably the most impactful as it can contribute significantly to the efforts to reduce greenhouse gas (GHG) emissions. Today, 95% of Riyadh’s residents use private cars [RUHV]. The introduction of metro services is expected to reduce that, but the impact may be less than hoped for, due to the last/first mile problem. By analyzing commute behaviors and door-to-door journey patterns, first/last mile journey challenges can be singled out and, therefore, better addressed, which may lead to increased uptake of metro services. To appreciate the potential impact, every 10% increase in metro uptake will equal a reduction of approximately 3 million tons of CO2 emissions in Riyadh [BNFT].

Another notable use case is LEAP, the Saudi Arabia’s global tech-event that has seen more than 100K+ visitors in 2023. However, the collective feedback on social media was that there was a significant amount of time spent moving into/out and within the exhibition floor. Any attempt from organizers to create a more seamless visitor experience would involve a lot of improvised effort based on anticipations unless visitor mobility data is measured. By only improving the last/first mile commute experience, the time spent by visitors can be boosted by more than 25%, based on a pilot project we carried out.
Challenges Ahead
In a very recent and insightful article, industry veterans point out a potential shortfall in Open RAN systems that could result in stifling innovation in the Open RAN domain [ODSL]. This is related to the limited coverage and breadth of KPMs (RAN data) available today in commercial Open RAN systems. In a recent study we commissioned from Kelley Business School on the addressable market opportunity that can be captured by open-loop is estimated near USD 10B [KLLY]. Given the massive opportunity that Open RAN offers under the closed-loop paradigm, this article is in essence calling for expedited improvement to RIC interfaces, namely: O1, E2, and the recently introduced Y1.
Other challenges include – from the point of view of mobile network operators:
- End-to-end (E2E) Integration: This challenge, due to multi-vendor ecosystem, include validations, version issues, interoperability, and feature parity, among others.
- Operational Challenges: Due to multi-vendor setup, a big challenge exist in how to ensure efficient conduction of tasks such as root cause analysis (RCA) and network issues accountability, inter-operability, regular software & patches upgrade in production issues.
- Cyber Security Challenges: Considering the regional regulator guidelines, adoption of open-source software issues, non-standardized or partial-standardized interfaces need to be critically examined, with particular emphasis on applicable data privacy and protection regulations.
- RIC & SMO Challenges: RIC & SMO automation layer is challenging, especially due to 3rd party xApps and rApps, interoperability with E2 nodes and multi-vendor components support.
Conclusions
Advocates of Open RAN have been so far primarily focused on the potential cost savings and supply chain diversification advantages it may bring to mobile operators. In this article, we rather shed light on the potential for Open RAN to unlock new value creation opportunities outside the network itself. We discuss in this article how Open RAN is well-positioned to address the growing needs of smart cities. Open RAN’s software-centric architecture lends itself to the exposure of various datasets and the application of AI workflows. With that kind of capability, a plethora of analytics and insights can be generated in the context of making our cities smarter and more cognitive.
References
- [SUSC] Dreaming of sustainable cities: from life goals to lifecycle analysis - KAUST Insight.
- [CAMC] Zizhu Fan, Hong Zhang, Zheng Zhang, Guangming Lu, Yudong Zhang, Yaowei Wang, A survey of crowd counting and density estimation based on convolutional neural network, Neuro-computing, Volume 472, 2022, Pages 224-251, ISSN 0925-2312.
- [WBLE] Laoudias, Christos, Adriano Moreira, Sunwoo Kim, Sangwoo Lee, Lauri Wirola, and Carlo Fischione. "A survey of enabling technologies for network localization, tracking, and navigation." IEEE Communications Surveys & Tutorials 20, no. 4 (2018): 3607-3644.
- [MAPP] Wang-Sub Lee, So Young You, Jin Ki Eom, Jiyoung Song, Jae Hong Min, Urban spatiotemporal analysis using mobile phone data: Case study of medium- and large-sized Korean cities, Habitat International, Volume 73, 2018, Pages 6-15, ISSN 0197-3975.
- [GHGC] Emissions of Carbon Dioxide in the Transportation Sector | Congressional Budget Office (cbo.gov).
- [CONN] Solutions to 10 Service Provider Pain Points (lightreading.com).
- [COVA] A flood of coronavirus apps are tracking us. Now it’s time to keep track of them. | MIT Technology Review.
- [LUCA] Lucas Bezerra, Nour Kouzayha, Hesham Elsawy, Ahmed Bader and Tareq Al-Naffouri, "CSI-Based Proximity Estimation: Data-Driven and Model-Based Approaches," in IEEE Open Journal of the Communications Society, vol. 5, pp. 97-111, 2024..
- [EAST] Mobile Networks Come to the Rescue of Brick-and-Mortar Businesses | Medium.
- [ODSL] Can Domain Specific Languages Pave the Open RAN Road to Success? | IEEE Communications Society (comsoc.org)
- [GCCO] Open RAN for Brownfield Operators, Challenges and Opportunities, GCC Open RAN Consortium Whitepaper, October 2022.
- [RUHV] Deloitte City Mobility Index 2020, Riyadh.
- https://www2.deloitte.com/content/dam/insights/us/articles/4331_Deloitte-City-Mobility-Index/Riyadh_GlobalCityMobility_WEB.pdf
- [BNFT] Environmental Benefits of Public Transit | The Environment.
- [KLLY] Strategic Market Evaluation for the Urban Mobility Segment, Deanna Deeter, Vladimir Ducasse, Ryan Glasgow, Austin Horbaly, and Kimberly Quintanilla, July 25, 2023.
- [NEOM] NEOM’S The Line: The world’s first cognitive city | WIRED Middle East.
Statements and opinions given in a work published by the IEEE or the IEEE Communications Society are the expressions of the author(s). Responsibility for the content of published articles rests upon the authors(s), not IEEE nor the IEEE Communications Society.