Hamid Jafarkhani, University of California, Irvine
Published: 25 Oct 2022

CTN Issue: October 2022
A note from the editor:
Climate change is fueling the frequency and intensity of wildfires, turning these natural phenomena into disasters of devastating proportions. In California, to name one specific region prone to wildfires, 2020 and 2021 saw the highest and second-highest numbers of acres burned in its historical record. This is therefore a problem of growing severity, and we engineers are in a position to be part of the solution. Indeed, the IEEE’s own motto is a constant reminder that our mission is to “advance technology for humanity,” and here is an opportunity for technology to come to the rescue on a matter of enormous importance. This month’s issue of CTN, authored by Prof. Hamid Jafarkhani from the University of California at Irvine, addresses the role that UAVs can play in the fight against wildfires, and how this role can be greatly enhanced by wireless communication and signal processing. It is an excellent review, and a call to arms for more research and development in this arena. Enjoy it.
Angel Lozano, CTN Editor
UAV Networks: A New Frontier in Fighting Wildfires

Introduction
The use of Unmanned Aerial Vehicles (UAVs) or drones in fighting wildfires is not an entirely new phenomenon [1]. Many fire departments use UAVs for different purposes in their operations. The highlight that caught people’s attention was the use of drones in the 2019’s Notre-Dame Cathedral fire. It was extensively reported by different news agencies that drone’s footage contributed to many tactical decisions and helped in stopping the Notre-Dame Cathedral fire and reducing the damage [2]. Currently, fire department agencies use UAVs equipped with photographic and infrared cameras for different goals, especially in initial wildfire localization, surveillance of the surrounding areas during the fire-fighting operation, visually inspecting hard-to-see places to understand the situation, and monitoring the fire progression.
As overall earth temperatures and droughts’ intensity and duration increase because of climate change, there is a dramatic swelling in the frequency and intensity of wildfires, both forest and structural fires. The result is a devastating effect on lives, property, and the environment. This calls for improving the science and engineering behind integration of UAVs in fighting wildfires. This is in line with recent efforts in integrating UAVs in wireless communication networks and contributions from the communications research community can have a huge impact. Currently, during fire-fighting operations, UAVs are mainly used individually, i.e., without connecting to each other to form a network. Their main role is in monitoring and surveillance using visual and infrared sensing. In most cases, they are operated manually to provide a better understanding of the fire’s surrounding areas or to monitor fire perimeter, inaccessible regions, and hot spots. UAVs primarily communicate, using unlicensed spectrum, to a screen that is viewed by the operator to visually monitor the scene.
It is not easy to operate UAVs, especially in the vicinity of the fire, because of the very high temperature. In fact, there have been incidents in which UAVs themselves were the cause of wildfires, like the Table Mountain Fire in Boulder County [3]. Some of the challenges in using UAVs for monitoring include the battery lifetime and subsequently the limited flight time, limited payload, and the manual coordination between the visual and infrared sensed data and other information available at the command-and-control center. Despite these limitations, UAVs can help in addressing unmet challenges. For example, UAVs can contribute to rapidly spotting vulnerable locations and in general providing situational awareness of wildfires at their earliest stages. They can be used in hard-to-reach areas that fire-fighters cannot access quickly. They can also supply information to assist better predictions of both local and event-scale behavior, better coordination of personnel and resources, and increased safety for fire-fighters on the ground. Their main advantage is their flexibility and the ease of deployment, especially flying under harsh conditions when pilot safety is a major concern. For example, at night or when thick smoke makes it dangerous for manned aircraft, UAVs equipped with multi-spectral cameras can provide useful information without risking the pilot’s life. Adding the fact that in remote areas and after disaster, existing communication infrastructure like cellular communication networks may not be available, there are many other important roles for a fleet of UAVs. For example, UAVs can provide a functioning communication and sensing network while operating as flying base stations (FBSs). In addition, UAVs can play a major part in collecting the necessary data to develop dynamic models for predicting fire behavior, for instance by monitoring the ember flux. The useful information that can be collected by UAVs include current weather and atmospheric conditions, terrain, and combustible fuel. To achieve these goals, a close collaboration among scientists and engineers with expertise in UAVs, wireless sensing, communication and network theory, multi-agent control, wildfire modeling, fluid mechanics, and atmospheric science is needed.
Opportunities and Challenges
Scientists study the wildfire behavior on different time scales and use a range of approaches from simple algebraic relationships relying on wind direction to very complex time-varying fluid dynamics models. Computational models are simulated to predict the overall direction and rate of fire spread, transitions in fire behavior, and recognizing fire’s characteristics [4]. The main challenge is the high complexity of the models that provide more accurate prediction and the real-time collection of the local data needed to improve the model’s prediction. Embers play a crucial role in scattering fire and igniting structures during an active wildfire. However, dynamic modeling of ember behavior is very hard and requires enormous data collection. In addition, it is very difficult to monitor new fire spots during a wildfire. To address these challenges, UAVs equipped with photographic, infrared, thermal, and multi-spectral cameras and lidar can collect important data that are not currently available. In fact, researchers already use UAVs to take footage of ember transport and forest floors during prescribed burns as shown in Figure 1. By comparing the images taken before, during, and after a prescribed forest burn, they can study the wildfire behavior and how it transforms the forest [5]. Incorporating data collected by UAVs into existing fire models can improve them dramatically. Ember tracking and short-term predictions of high-risk areas play important roles in fire-fighting.

As the use of UAVs in fire-fighting expands, there is the need for automatically, or semiautomatically, deploying UAVs in the areas of interest. Also, maintaining a connected network of UAVs is essential for achieving the goals discussed earlier. Optimizing UAV trajectories during the operation to perform certain tasks while communicating with the command-and-control center and fire-fighters on the ground is an important challenge. There has been much effort in developing different techniques for UAV trajectory optimization and optimal UAV deployment in the communication literature [6]–[13]. These optimal trajectory and deployment algorithms consider complicated RF models for directional antennas, interference, realistic throughput calculation, delay, UAV energy consumption models, including flying energy with mechanical constraints, obstacle and collision avoidance, and battery life to name a few. The corresponding sophisticated techniques developed for the purpose of optimizing the desired utility functions, say maximizing the communication throughput, under practical constraints (power, coverage, delay, and so on) can be adopted to address UAV trajectory optimization and deployment for fire-fighting. It is time to bring wildfire related objectives and constraints into the formulation. An automatic UAV deployment system needs to incorporate priorities like fire localization, prediction time scales, and communications requirements from the command-and-control center and the fire-fighters. They also need to use a priori information like coarse initial fire location, inhabited areas, and infrastructure.
Understanding the opportunities and challenges of integrating UAVs in wildfire modeling, prediction, and management can have a huge impact in fighting wildfires. There is a need to design a network of UAVs and wireless sensors that can participate in initial wildfire localization, mapping the affected areas, and near-term prediction of fire progression, as discussed before. Simultaneously, there is an effort within the research community and the international standardization bodies to utilize UAVs for augmenting the existing wireless communication networks [15]. In fact, in addition to serving UAVs as users of existing wireless communication networks, UAVs can help wireless systems by acting as FBSs for cellular networks, access points for sensor networks, and relays [16]. They can create a flying wireless ad-hoc network or even connect to satellite communication. They can use reconfigurable intelligent surfaces (RIS) on the ground or carry RIS to help covering blind spots on the ground. Figure 2 shows different example roles that UAVs can play in future wireless communication and sensing networks.
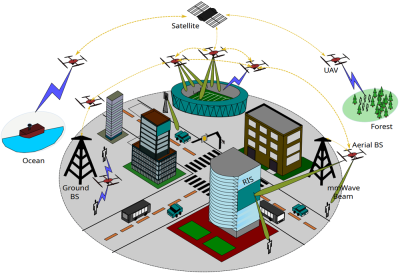
While a high vantage point results in many opportunities for Line of Sight (LoS) communication, which results in better performance for the targeted transceivers, it also creates many opportunities for unintentional interference that, if left unchecked, may limit, rather than enhancing the operation, ruining the promising potentials of UAVs in improving the performance of the wireless networks. While one of the main spectral efficiency characteristics of a cellular network is the frequency reuse in different cells, UAVs may simultaneously and unintentionally interfere with other UAVs and users in neighboring and even faraway cells because of their altitude and the strength of the LoS channels. Therefore, managing the interference caused by UAVs to users in other cells is an important research challenge. How to deal with interference in UAV communication and sensing networks and the appropriate UAV network architectures during wildfires are open research challenges.
Applications of using UAVs to augment existing wireless communication networks include cost-effective service in hard-to-reach rural areas and on-demand load-relief in existing terrestrial networks, among others. FBSs embodied by UAVs are attractive solutions for collecting and transmitting data in real-time, for example to support sensing and telemetry applications. Also, FBSs provide superior coverage and can be deployed quickly and on-demand, for example to relief the load in existing terrestrial networks. This latter aspect is especially relevant in fighting wildfires when the fixed infrastructure is damaged or outright non-existent. The two efforts, i.e., integrating UAVs in fighting wildfires and utilizing UAVs for augmenting the existing wireless communication networks, can help and influence each other. While there are some fundamental differences, many technical challenges and the relevant tools to address them are similar. This opens the door for communication theorists and practitioners to contribute to the important challenge of fighting wildfires.
References
- “Drones are Playing an Increasingly Important Role in Fighting Wildfires,” https://wildfiretoday.com/2022/10/05/drones-are-playing-an-increasingly-important-role-in-fighting-wildfires/.
- “Notre Dame Fire Chief: ‘The Roof Was Lost, We Had to Save the Belfries’ ,” https://www.thetimes.co.uk/article/a300b520-608b-11e9-9842-63958b78574e.
- “Drone Crash Blamed For Table Mountain Fire In Boulder County,” https://www.cbsnews.com/colorado/news/table-mountain-fire-boulder-county-drone-crash/.
- J. Coen, W. Schroeder, and B. Quayle, “The Generation and Forecast of Extreme Winds during the Origin and Progression of the 2017 Tubbs Fire,” Atmosphere, vol. 9, p. 462, 2018.
- “How to Save a Forest by Burning it,” https://www.nytimes.com/2022/09/07/climate/california-wildfire-prescribed-burn.html.
- E. Koyuncu, M. Shabanighazikelayeh, and H. Seferoglu, “Deployment and Trajectory Optimization of UAVs: A Quantization Theory Approach,” IEEE Trans. on Wireless Commun., vol. 17, pp. 8531–8546, Dec. 2018.
- C. Diaz-Vilor and H. Jafarkhani, “Optimal 3D-UAV Trajectory and Resource Allocation of DL UAV-GE Links with Directional Antennas,” 2020 IEEE Global Commun. Conf., pp. 1–6, Dec. 2020.
- M. Alzenad, A. El-Keyi, F. Lagum, and H. Yanikomeroglu, “3-D Placement of an Unmanned Aerial Vehicle Base Station (UAV-BS) for Energy-Efficient Maximal Coverage,” IEEE Wireless Commun. Lett., vol. 6, pp. 434–437, Aug. 2017.
- Q. Wu, Y. Zeng, and R. Zhang, “Joint Trajectory and Communication Design for Multi-UAV Enabled Wireless Networks,” IEEE Trans. Wireless Commun., vol. 17, pp. 2109–2121, Mar. 2018.
- G. Zhang, H. Yan, Y. Zeng, M. Cui, and Y. Liu, “Trajectory Optimization and Power Allocation for Multi-Hop UAV Relaying Communications,” IEEE Access, vol. 6, pp. 48566–48576, Aug. 2018.
- S. Zhang, H. Zhang, Q. He, K. Bian, and L. Song, “Joint Trajectory and Power Optimization for UAV Relay Networks,” IEEE Commun. Lett., vol. 22, pp. 161–164, Jan. 2018.
- F. Cheng, S. Zhang, Z. Li, Y. Chen, N. Zhao, F. R. Yu, and V. C. Leung, “UAV Trajectory Optimization for Data Offloading at the Edge of Multiple Cells,” IEEE Trans. Vehicular Technology, vol. 67, pp. 6732–6736, Jul. 2018.
- C. Diaz-Vilor, A. Lozano, and H. Jafarkhani, “On the Deployment Problem in Cell-Free UAV Networks,” 2021 IEEE Global Commun. Conf., pp. 1–6, Dec. 2021.
- “SMAC-FIRE: Closed-Loop Sensing, Modeling and Communications for WildFIRE,” https://nsf.gov/awardsearch/showAward?AWD_ID=2209695.
- 6th ICC Workshop on Integrating UAVs into 5G and Beyond, May 2023.
- Y. Zeng, Q. Wu, and R. Zhang, “Accessing From the Sky: A Tutorial on UAV Communications for 5G and Beyond,” Proc. of the IEEE, vol. 107, pp. 2327–2375, Dec. 2019.
This work was supported in part by NSF Award CNS-2209695. The author is the director of the Center for Pervasive Communications and Computing, Univ. of California, Irvine. Email: hamidj at uci.edu
Statements and opinions given in a work published by the IEEE or the IEEE Communications Society are the expressions of the author(s). Responsibility for the content of published articles rests upon the authors(s), not IEEE nor the IEEE Communications Society.